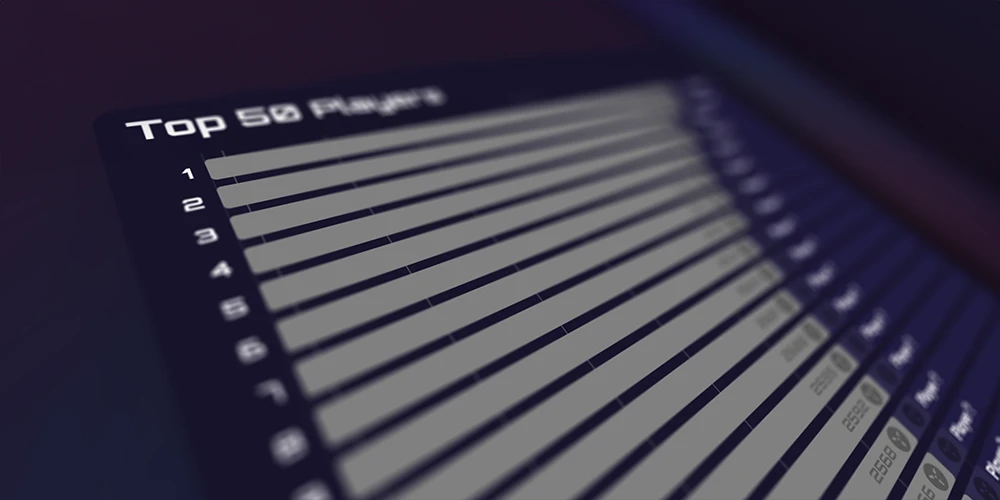
Top 50 Players of the RLCS 2022/23 Season
This year's RLCS season was the biggest ever. The changing times have forced many of the old guard into retirement while the youngsters take over the LAN events. The RLCS schedule was also retired after this season, and we'll have to wait until next year to see how it will continue. But, the long offseason is the perfect time to reflect on the past season and crown the best players of the season as a whole.
The methodology
As per usual, the rankings will be based on my custom AI system (learn about it here). However, there is a major difference to the standard daily rankings on rocketscience.fyi. The rating system has a recency bias built in by default. Here is why: Any time a player wins or loses against a team, their rating change depends on the relative rating difference to that team. If a player goes on a loss streak and ends up with a really low rating, they will from now on be earning more points for each win than if they hadn't lost. This allows them to recover back to their deserved rating quicker, but it also means that it matters much more how you recover than how you started: recency bias.
That's perfect for daily rankings, but if I were to use that idea for the entire season, winning the Fall Major 2022 would essentially not matter for the final position in the ranking. The solution: rating periods. By using a rating period, you can essentially pretend that all games in the rating period happened at the same time, completely eliminating recency bias. Sounds great; however, that also means that a team's rating won't change at all between different matches of the same rating period. We all know that teams don't have the same strength throughout the entire season, so it wouldn't be very accurate. So I went with a hybrid approach instead. First, I run everything without rating periods, collecting all the necessary data to estimate the varying strength of teams at the time of each game. Then I reset all the players to the same start and feed all the games into one rating period (taking into account the variable team strength).
Games & weighting
The dataset for the rating period contains all RLCS tournaments of the season, no third-party events.
LANs are generally regarded as more important than online play. Usually, I don't care about that because my goal is to predict results as well as I can, and online performance has proven to be (on average) just as indicative of future results as LAN matches. performing on LAN (specifically) is not a reliable predictor of skill. However, for the purpose of giving out these imaginary trophies, I've decided to weight the results of the Majors at 3x the strength of a regional. This also means that all 3 regionals of the split combined are worth as much as the one Major. The World Championship gets an extra 50% importance on top, ending up with a 4.5x multiplier.
And that leads us to yet another issue: Not all players play in every tournament. That is not an issue in itself, but the problem is that some players might get punished for qualifying and then losing at a tournament with tougher competition. The players that didn't manage to qualify would've likely done as bad or worse. In order to overcome this issue, I considered a couple of options and ultimately decided on the following: Every player that fails to qualify for a Regional/Major/Worlds effectively loses 2 fake series against a fake team that matches the average skill at the event. Why 2? First of all, I didn't want to separate my code between the different splits, and in Spring, you're out after 0-2. Secondly, evaluating games that didn't really happen always has downsides. You're punishing someone for something they didn't do. You never know what result they would've actually gotten if had managed to qualify. The teams that didn't qualify will already have worse records anyways, so 2 losses will likely be more than enough to keep them below the teams that really got a shot at Regionals/Majors/Worlds.
Yes, I did account for players like zen, oaly, Aztral, and Extra. They were not punished for being unable to participate due to reasons outside of their control.